Why Pretraining is key to intelligence and bittensor
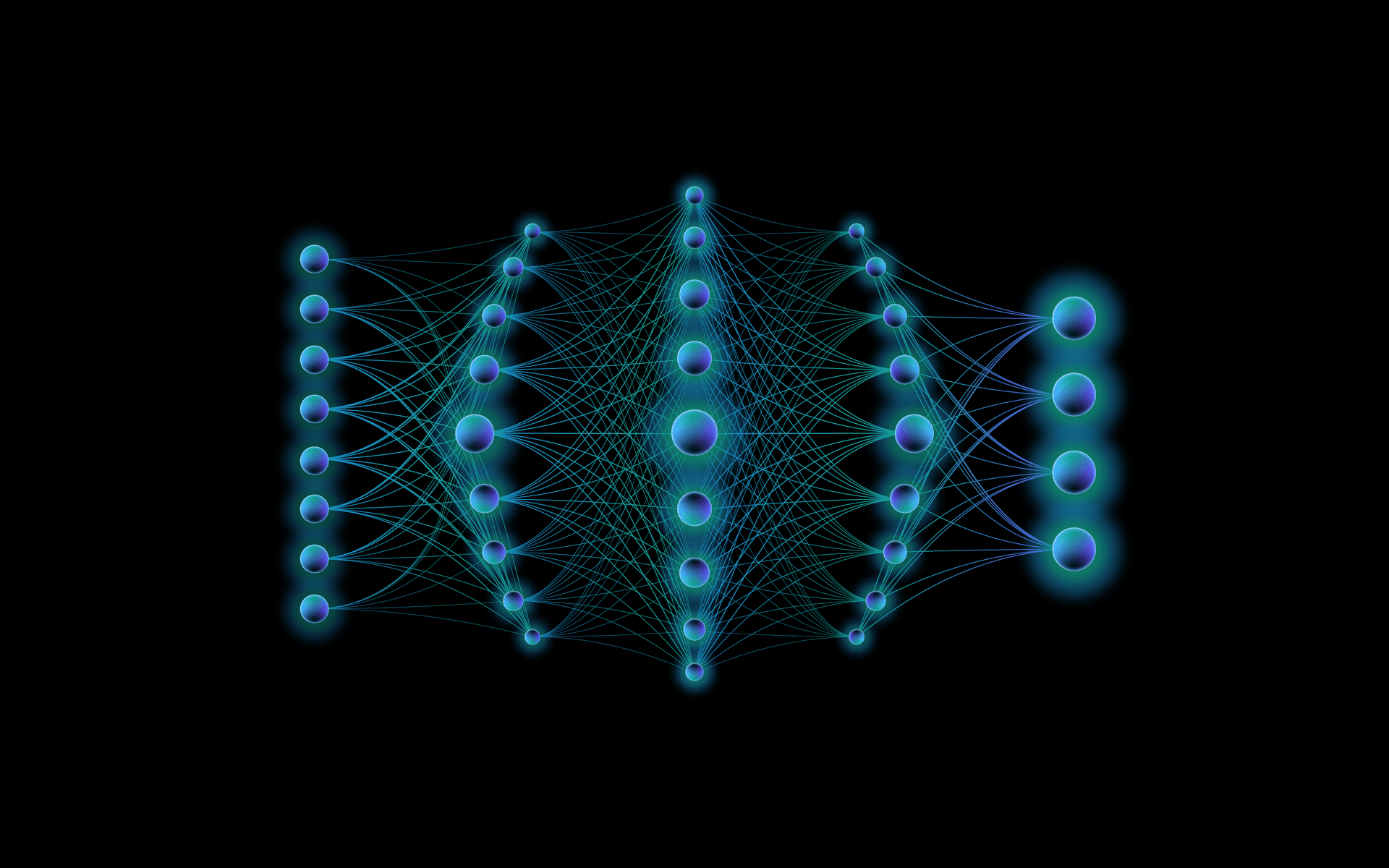
SN9 is Bittensor's pre-training subnet, managed by Macrocosmos
It's designed to provide pre-trained models to the Bittensor ecosystem, incentivising miners to build the best models.
For LLMs, the design choices, training methods and training data shape outcomes
In centralised, closed-source companies, pretraining is a closely guarded secret, shaped by the priorities of the organization. Leaders spend huge amounts of time, money and compute to pretrain a model before fine-tuning it for a specific use case.
Pretraining represents a valuable use case for the power of the Bittensor
Marginal improvements to pre-training can have significant downstream benefits for subsequent AI models built within Bittensor, because it provides the underlying foundation work and raw intelligence that can power individual use cases across multiple subnets.
How SN9 trains SOTA Intelligence
Incentivising quality output
SN9 incentivises miners to provide the highest quality pre-training models and validators that assess the quality of the miner's models. Quality is assessed by the validators against a standard agreed by the community.
Identify AND reward contributions
A winner-takes-all model stimulates minors to continuously compete, so that their model has the best chance of being number one. This system encourages miners to commit resources and compute power.
De-risk influence of bad actors
The other consequence of a winner-takes-all compensation structure is that it encourages greater professionalism among miners, while discouraging cabbalistic behavior. Together with robust validation mechanisms, SN9's design ensures that it pays to play by the rules.