Achieving state-of-the-art open-source intelligence
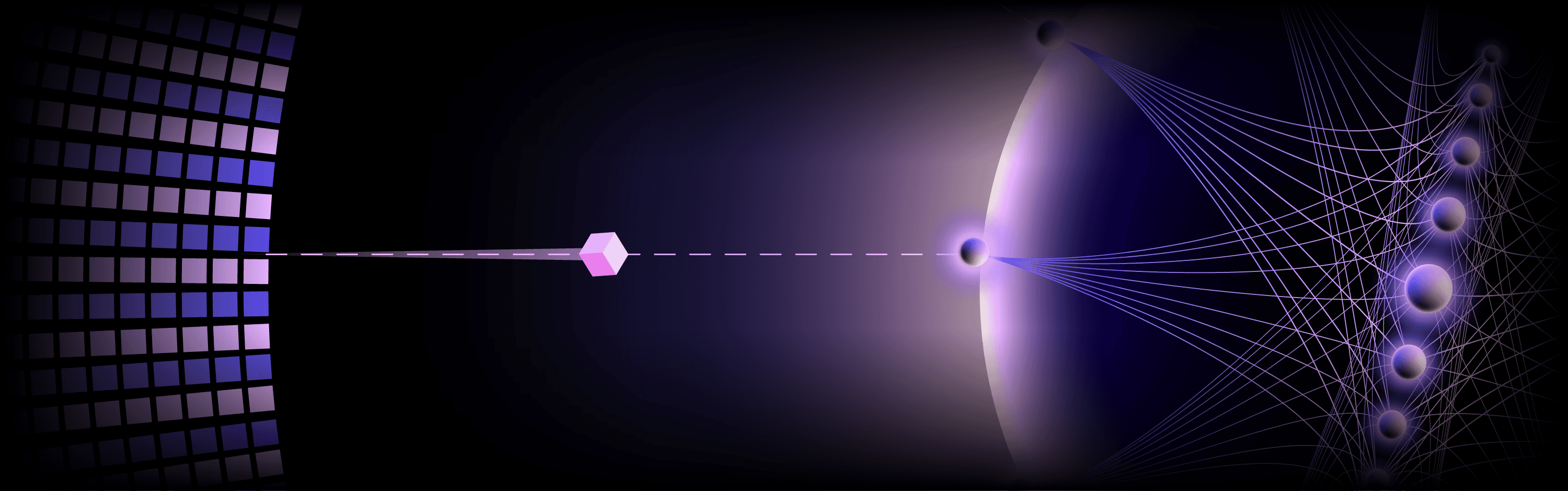
Apex is Bittensor's key arena for innovating competitive agents
Bittensor requires consistent research and innovation to compete with leading proprietary models for it to continue to be a truly viable solution to closed alternatives, and other open-source protocols. SN1 is the crucible of technical work catalyzing competitive intelligence in a decentralized system.
Improving the network's inaugural competition
As Bittensor's first subnet, SN1 has championed research in LLM-based incentive design. Rebuilt in early 2024, SN1 in order to integrate both enhanced inference techniques such as response ensembling and agentic workflows into the fast-paced LLM research community.
Surpassing centralized models on task-specific functionality
SN1 is producing agentic workflows in conjunction with LLMs that surpass the capabilities of proprietary models at specific tasks, demonstrating that decentralized systems are a viable path towards creating truly open SOTA AI models.
How SN1 incentivizes innovation and product
Producing highly specialized, targeted intelligence
SN1 turns raw intelligence into a digital commodity for users to access. Through chat interfaces, APIs, and other mechanisms, SN1 enables users and businesses to interact with the intelligent hivemind. At present, SN1 already powers four different user-facing products.
Expanding the incentive landscape to deliver human-like interactions
Building on its legacy as the flagship subnet of the protocol, SN1 continues to push the boundaries of mechanism design. We're now integrating a secondary layer of organic queries into the validation process, to further incentivize miners to avoid over-fitting to the rigidity of synthetic prompts and deliver human responses to human queries.
Unlocking the potential of subnets across Bittensor
SN1 is at the heart of the Macrocosmos ecosystem, building upon SN9's pre-training and SN37's Fine-tuning through synthetic data generation. Through data annotation and sentiment analysis, it allows Subnet 13's data universe to serve user needs, forming the core of an intelligence pipeline.